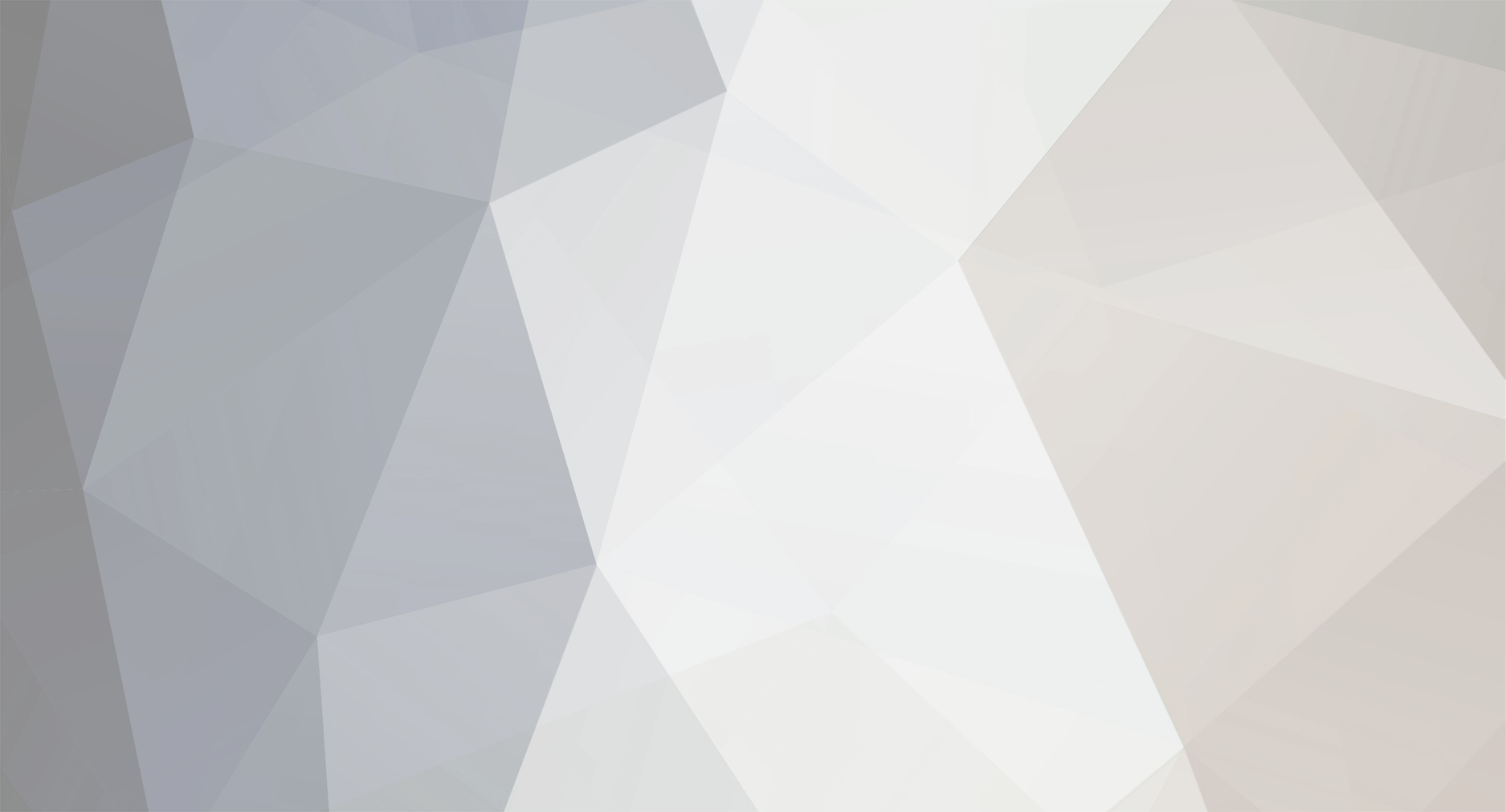
TheClimateChanger
Members-
Posts
3,124 -
Joined
-
Last visited
About TheClimateChanger

Recent Profile Visitors
The recent visitors block is disabled and is not being shown to other users.
-
Looking solid for us. Kind of on the tripoint between broiling with average precipitation, sultry and thunder-filled, and brutally humid and wet.
-
-
This is interesting. If snowfall continues dropping at the average rate of the 21st century, it will drop below -20" [negative 20 inches] by 2050 at Cleveland.
-
Occasional Thoughts on Climate Change
TheClimateChanger replied to donsutherland1's topic in Climate Change
This was also funny. Some "skeptics" allegedly used Grok to co-author a paper critical of AGW to publish in a bogus journal, and Grok rips the paper to shreds and denies he authored it. -
Occasional Thoughts on Climate Change
TheClimateChanger replied to donsutherland1's topic in Climate Change
You may be right. I see graphs posted all the time like the one Zeke addressed, and they seem to ignore the elephant in the room that, in the vast majority of time, temperatures were much warmer, but so was CO2. And temperatures were much warmer despite lower solar output. The "adjustment" arguments are just as ridiculous. How do these people explain decreases in ice cover, phenological changes, receding glaciers, increases in sea and lake temperatures, etc. all consistent with warming? Like, if the warming is due to adjustments, what the heck is causing those changes? How about the fact that satellite analyses largely confirm the trend since 1979, and radiosondes even longer? Gold standard USCRN stations are in lock step with NOAA's nClimDiv dataset since 2005 (actually slightly larger warming trend)? -
Occasional Thoughts on Climate Change
TheClimateChanger replied to donsutherland1's topic in Climate Change
-
Wow, I just noticed PIT had a gust to 54 mph at the top of the hour, without a wind advisory or even a special weather statement. I guess there is a hazardous weather outlook which notes gusts of up to 54 mph.
-
Occasional Thoughts on Climate Change
TheClimateChanger replied to donsutherland1's topic in Climate Change
I mean over geologic time scales, not in the near future. CO2 levels have largely been declining naturally since the Paleocene, due to weathering and decreased volcanism/geological activity as the earth's core settles over time. Over that same interval, the climate has cooled naturally in response to the decrease in CO2 with ice ages commencing around 2.58 mya which have gradually become longer and harsher over the course of the Pleistocene. These trends would very likely continue indefinitely into the future if there was no massive release of stored carbon from human activity, until solar irradiance increased sufficiently to reverse that trend. -
Occasional Thoughts on Climate Change
TheClimateChanger replied to donsutherland1's topic in Climate Change
I ran it by Grok and he agreed with my theory, albeit maybe not to the extremes I posited. He suggests colder and longer lasting ice ages for up to the next 10 mya [again, in the absence of human activity], but nothing suggesting a total loss of the interglacial cycle. -
Occasional Thoughts on Climate Change
TheClimateChanger replied to donsutherland1's topic in Climate Change
Do you think in the absence of human activity, the planet would be destined for a near-permanent ice age in the geological future? Obviously, such an ice age could not truly be permanent, because solar irradiance is increasing over time as the sun is a main sequence star. In the very distant future, this will inevitably lead to runaway warming. But in the nearer term (next few millions of years), it seems likely that ice age conditions would become the norm. In millions of years, could the earth go from near snowball earth conditions to typical quaternary ice age conditions from Milankovitch cycles [rather than from ice age to interglacial]. Looking at the geological record, we can see, generally, a long-term cooling since the Paleocene, which coincides with a long-term decrease in carbon dioxide. This appears to be due to increased weathering and a decrease in volcanism over time. As the earth continues to age, there would continue to be fewer and fewer volcanic eruptions over time, which, in the absence of human activity, would result in continued decreases in carbon dioxide. I wonder if carbon dioxide levels would eventually drop so low that photosynthesis might cease. It looks as though the planetary trajectory is towards extreme cold and eventually extreme heat. -
Occasional Thoughts on Climate Change
TheClimateChanger replied to donsutherland1's topic in Climate Change
There are also statistical methods to correct for inhomogeneities that don't involve any explicit adjustments (kriging, pairwise homogenization). -
Occasional Thoughts on Climate Change
TheClimateChanger replied to donsutherland1's topic in Climate Change
But they can? You can just set up a controlled experiment to compare the two and determine the net bias? No need for any time travel! -
Occasional Thoughts on Climate Change
TheClimateChanger replied to donsutherland1's topic in Climate Change
And that's another reason they love the Tmax charts. They get to ignore the cooling bias of Tmax occasioned by the switch to MMTS from CRS. You guys are the real climate hoaxers! -
Occasional Thoughts on Climate Change
TheClimateChanger replied to donsutherland1's topic in Climate Change
Why don't you read a history book? Most of the old temperature records were compiled from rooftop stations in cities, often near chimneys and such, and in the late 1800s, the thermometers were sometimes housed on balconies or window cavities. I bet that automated sensor has more of a cooling bias relative to a LiG thermometer housed in a cotton-region shelter [aka Stevenson screen] than any warming bias from the surroundings. -
Occasional Thoughts on Climate Change
TheClimateChanger replied to donsutherland1's topic in Climate Change
Always the raw Tmax temperatures so they can ignore the warming bias of resetting at 5 or 6 in the afternoon in the olden days. I'm gonna guess the Tmin temperatures don't show the same trend, and Tavg in between. Also, they typically don't even bother to grid these data [which would account for changes in elevation and geospacial siting of the stations] but rather just average all of the data. Sorry, can't do that!