-
Posts
440 -
Joined
-
Last visited
About MegaMike
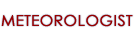
- Birthday 09/09/1993
Profile Information
-
Four Letter Airport Code For Weather Obs (Such as KDCA)
KOWD
-
Gender
Male
-
Location:
Wrentham, MA
Recent Profile Visitors
The recent visitors block is disabled and is not being shown to other users.
-
It's an experimental model that doesn't predict moisture/heat flux based on fluid dynamics. As a result, it's totally unreliable in my opinion... It's probably the reason why the NWS doesn't refer to it in their forecast discussions. Can't wait to see how it performs during the warm season. With limited training data on hurricanes, I expect it to perform terribly with tropical disturbances.
-
I agree with you both. To evaluate snowfall, you really need to evaluate SWE, as well. For that matter, you'd need to evaluate forcing fields too (ensure SWE was predicted accurately for the right reasons). If SWE was under predicted, but a snowfall algorithm performed well, that algorithm isn't showing accuracy... It's showing a bias. Unfortunately, snowfall evaluations are tricky because of gauge losses wrt observations. Not everyone measures the same either... Can of warms, snowfall is. A met mentioned this earlier too, but the more dynamic an algorithm is, the more likely errors exacerbate. The Cobb algorithm is logically ideal for snowfall prediction, but compounding error throughout all vertical layers of atmosphere likely inhibits its accuracy. Snowfall prediction sucks which is probably why there are only a handful of publications. Otherwise, these vague algorithms wouldn't be widely used by public vendors.... It's the bottom of a very small barrel.
-
I've never heard of Spire Weather before, but based on their website, it looks like it's another AI modeling system (someone correct me if I'm wrong). It doesn't take much to run an AI model... Especially since the source code for panguweather, fourcastnet, and graphcast are available online for free: https://github.com/ecmwf-lab/ai-models My recommendation: If they don't evaluate or provide modeling specifications, don't use it.
-
I'm not sure if you're being sarcastic, but please don't use the CFS for this. The CFS is a heavily truncated (low horizontal, vertical, and temporal resolution) modeling system solely intended for climate forecasting. It won't perform well with a dynamic beast (which may or may not occur).
-
I have a hard time trusting the NAM 3km due to an issue (possibly patched or related to a vendor?) caused by its domain configuration and dynamics/physics options... If I remember correctly, if a large-scale disturbance moved too quickly, a subroutine will sporadically calculate an unrealistic wind speed (only aloft) at certain sigma levels... Can't predict atmospheric flux if a forcing field is kaput. I need to find this case study... It's pretty interesting and it happened twice from ~2016-2020. The erratic nature of NAM is off-putting too, but I'm thinking that's related to its ic/bcs... Before a system materializes, you're solely relying on ic/bcs from a regional modeling system. Those regional modeling systems cannot effectively predict, or even initialize, small-scale/convective features which leads to significant error over time. The same forecast volatility will likely occur to other mesoscale modeling systems if they ran past 48 hours. While on topic: I would like to see vendors start using the RRFS (https://rapidrefresh.noaa.gov/RRFS/; currently under development)... especially for snowfall and precipitation forecasting. It's a unified, high resolution modeling system which runs every hour. Consider it like the GEFS, but with the HRRR. The NBM/HREF are great, but its a waste of resources to post-process different mesoscale modeling systems onto a constant grid. Let's just be happy nobody mentioned the NOGAPS. Also not a fan of the ECMWF AI... That model is for data scientists, not meteorologists.
-
At this time, nobody should be relying too heavily on global (GFS, CMC, ECMWF) or regional (RGEM 10km, RAP 13km, NAM 12km, etc...) modeling systems. Stick with the mesos (HRRR 3km, WRF ARW, WRF ARW2, NAM 3km, etc...) and rely on data assimilation/nowcasting to follow any trends. That said, the 12z HREF (ensemble of mesos) increased mean snowfall for most areas in eastern MA and all of RI (compared to last night at 00z). It also expands accumulating snowfall westward.... More snow may be falling after this, but I'm too impatient to wait for the next panel... Follow it here: https://www.spc.noaa.gov/exper/href/?model=href&product=snowfall_024h_mean§or=ne&rd=20241220&rt=1200
-
December 2024 - Best look to an early December pattern in many a year!
MegaMike replied to FXWX's topic in New England
Good eye/question! The most recent version of the GFS has 127 hybrid sigma-pressure (terrain following at the surface and pressure aloft) vertical profile layers. In the plot you attached, the vendor is/appears to be plotting vertical profile data that has been interpolated to mandatory isobaric surfaces (1000, 925, 850, 700, 500, 400, 300, 250, 200, 150, and 100 mb). I'm assuming the same vendor uses a diagnostic categorical precipitation type/intensity field to plot precipitation type/intensity, which considers all 127 native vertical profile layers. So the Skew-T is only showing you a select few isobaric surfaces although the native model output possesses plenty more. -
I published a paper for the EPA regarding an air quality modeling system/data truncation, but I haven't collaborated with NCEP on atmospheric models/simulations. I do have experience with a bunch of different modeling systems though (in order): ADCIRC (storm surge), SWAN (wave height), WRF-ARW (atmospheric and air quality), UPP (atmospheric post-processor), CMAQ (air quality), ISAM (air quality partitioning), RAMS ( atmospheric), ICLAMS (legacy atmospheric model).
-
The HREF is a Frankenstein model since it requires post-processing to obtain fields on a constant grid. It's not a standard modeling system like the HRRR, NAM, GFS, etc... NWP requires a lot of static/time varying fields to run atmospheric simulations: vegetation type, elevation height, ice coverage, etc... By interpolating data onto a grid (or even transforming a horizontal datum), you degrade model accuracy since this information is lost. The HREF exists simply because we created a high-resolution ensemble with what we currently have.
-
Consider the RRFS an ensemble consisting of multiple (9 members + 1 deterministic) high-resolution (3km) simulations on a constant grid (unlike the HREF and NBM). In other words, it's similar to the HREF, but without any post-processing... The HREF requires post-processing since its ensemble members have various domain configurations (which is a bit taboo). Based on < https://gsl.noaa.gov/focus-areas/unified_forecast_system/rrfs >, it's set to replace the NAMnest, HRRR, HiResWindows, and HREF modeling systems. Besides what I mentioned above, the lateral boundary conditions come from the GFS (control) and the GEFS (members). All share the same core (FV3). Currently, they're testing a bunch of different options to improve the initial conditions (data assimilation) of the RRFS. Overall, it sounds like it'll be a significant improvement over the HREF and NBM once the RRFS becomes operational (and well tested). Note: I can't find much on the RRFS' performance... Based on what I've seen so far, It performs better in terms of reflectivity detection < https://www.spc.noaa.gov/publications/vancil/rrfs-hwt.pdf >
-
You don't think our modeling systems are trusted? Most high-resolution modeling systems perform well within 12 hours (especially once a disturbance is properly assimilated). Additionally, most global models perform reasonably well within 4 days at the synoptic level. If you expect complete accuracy for moisture/precipitation fields, you (not you specifically, just in general) don't understand the limitations of NWP... Our initial conditions/data assimilation, boundary conditions, parameterizations, and truncations (dx,dy,dz increments) leads to significant error over time which aren't necessarily related to a modeling system itself. If we could perfectly initialize a modeling system, theoretically, there would be little to no errors post-initialization. You can't say the same thing about an AI model since it's likely trained on forcing variables such as temperature/moisture (at the surface and aloft) and is not simulated using governing equations and fundamental laws which the atmosphere adheres to. I wrote, 'AI can be used to improve the accuracy of NWP output that have a known, and predictable bias...' so if we used AI to correct singular fields prior to initialization and while a modeling system is running, sure... It will likely improve the accuracy of NWP. Bottom line; use AI to assist NWP or to correct fields with known biases. At this time, I don't trust atmospheric, AI models.
- 1,593 replies
-
- 2
-
-
-
I'm not sure why anyone would use AI to forecast the weather. 1) AI is a glorified analog so it won't perform well for anomalous events. 2) We don't know what's incorporated in AI models so we can't correct for known biases. 3) Why use AI when we already have trusted NWP models? AI can be used to improve the accuracy of NWP output that have a known, and predictable bias though. For example, improving wind speed: < https://agupubs.onlinelibrary.wiley.com/doi/full/10.1029/2018JD029590 >.
- 1,593 replies
-
- 4
-
-